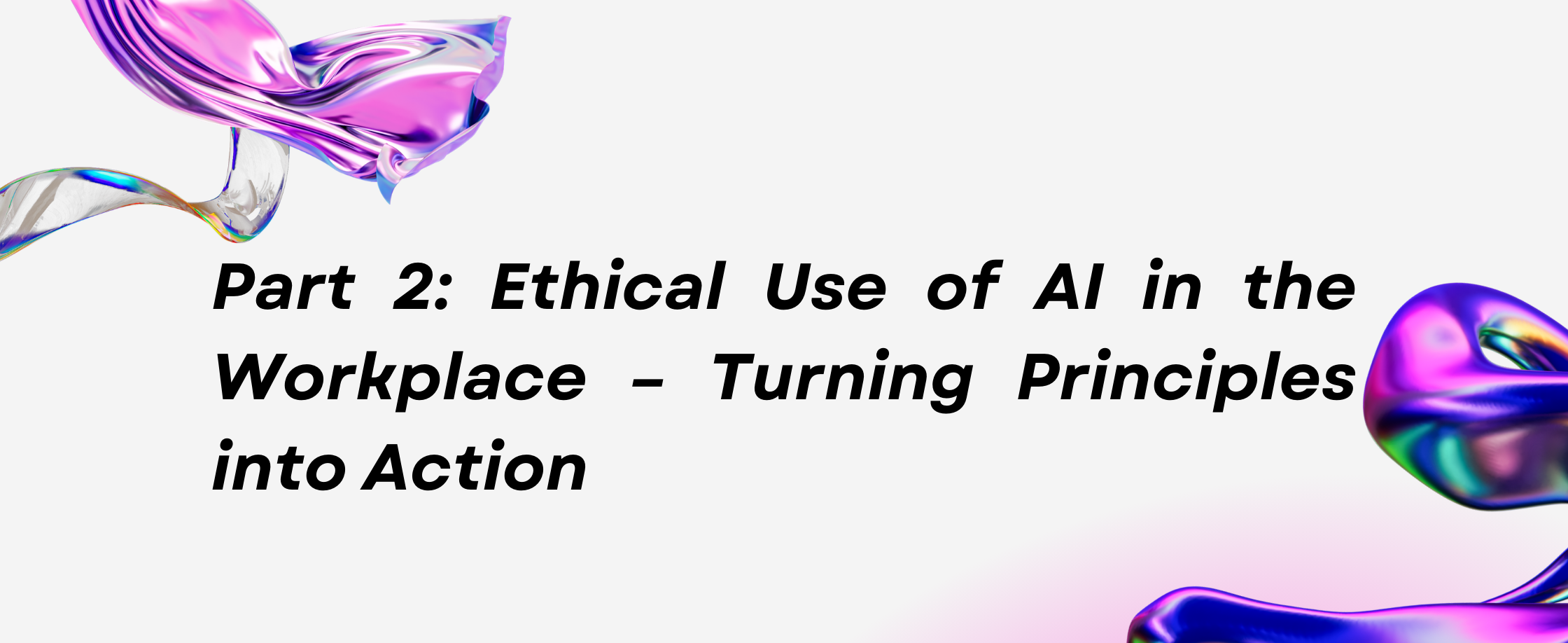
Introduction
In Part 1, we explored the why behind ethical AI in the workplace—building trust, equity, and accountability. But how do we turn these principles into actionable steps that drive real change? Let’s dive deeper into practical strategies organizations can implement to ensure AI works for everyone, not just a select few.
1. From Transparency to Empowerment
Why it matters: Transparency isn’t just about sharing data—it’s about empowering employees to understand and question AI decisions.
Action steps:
-
AI Literacy Programs: Launch workshops to teach employees how AI works. For example, explain how a hiring algorithm scores resumes or how a productivity tool tracks performance.
-
Interactive Dashboards: Create user-friendly dashboards that show how AI models make decisions. Think of it as a “behind-the-scenes” tour of your AI systems.
-
Feedback Loops: Encourage employees to flag unclear AI decisions. For instance, if an AI tool recommends a promotion, employees should know why.
2. Trust Through Collaboration
Why it matters: Trust isn’t built overnight—it’s earned through consistent communication and collaboration.
Action steps:
-
Co-Creation with Employees: Involve employees in designing AI tools. For example, when implementing an AI scheduling system, ask shift workers for input to ensure it meets their needs.
-
Ethics Champions: Appoint team members as “AI Ethics Champions” to advocate for fairness and address concerns.
-
Real-Time Updates: Use internal newsletters or Slack channels to share updates on AI projects, challenges, and successes.
3. Tackling Bias Head-On
Why it matters: Bias in AI isn’t just a technical issue—it’s a human one. Addressing it requires a proactive, multi-layered approach.
Action steps:
-
Bias Audits: Regularly test AI systems for bias using tools like IBM Fairness 360 or Google’s What-If Tool. For example, check if your hiring algorithm favors certain universities or genders.
-
Diverse Data Sets: Ensure your training data reflects the diversity of your workforce and customers. For instance, if you’re building a voice recognition tool, include accents from different regions.
-
Third-Party Reviews: Partner with external experts to audit your AI systems and provide unbiased feedback.
4. Inclusive Design: Beyond Tokenism
Why it matters: Inclusive design ensures AI tools work for everyone, not just the majority.
Action steps:
-
Employee Panels: Create focus groups with employees from diverse backgrounds to test AI tools before rollout. For example, involve employees with disabilities when testing accessibility features.
-
Localized Solutions: Tailor AI tools to fit local contexts. For instance, if you’re rolling out an AI chatbot in Nigeria, ensure it understands Nigerian English and cultural nuances.
-
Iterative Feedback: Continuously improve AI tools based on employee feedback. Think of it as a “living, breathing” system that evolves with your workforce.
5. Ethics as a Core Value
Why it matters: Ethical AI isn’t a one-time project—it’s a mindset that should permeate your organization.
Action steps:
-
Ethics Training: Mandate AI ethics training for all employees, from developers to HR. For example, teach teams how to identify and mitigate bias in data.
-
AI Ethics Officers: Hire or appoint dedicated roles to oversee ethical AI practices. These officers can act as a bridge between leadership, employees, and AI systems.
-
Public Commitments: Publish your organization’s AI ethics principles and progress reports. Transparency builds trust with both employees and customers.
6. Measuring Success: Beyond the Numbers
Why it matters: What gets measured gets managed. But ethical AI requires more than just metrics—it requires a commitment to continuous improvement.
Action steps:
-
Employee Surveys: Regularly survey employees to gauge their trust in AI systems. For example, ask if they feel AI tools are fair and transparent.
-
Impact Assessments: Measure the real-world impact of AI decisions. For instance, track whether AI-driven promotions lead to more diverse leadership teams.
-
Iterative Improvements: Use feedback and metrics to refine AI systems. Think of it as a cycle of learning and growth.
Conclusion: Ethical AI is a Journey, Not a Destination
Ethical AI isn’t about perfection—it’s about progress. By embedding transparency, collaboration, and inclusivity into your AI strategy, you can build systems that empower employees, foster trust, and drive innovation.
What steps is your organization taking to ensure ethical AI? Share your thoughts in the comments—let’s keep the conversation going on LinkeIn!
Let’s make AI work for everyone—ethically, inclusively, and transparently!